How to Set Up A/B Testing for Your Online Business: A Comprehensive Guide
- Danilo Stern-Sapad
- Marketing
- June 26, 2023
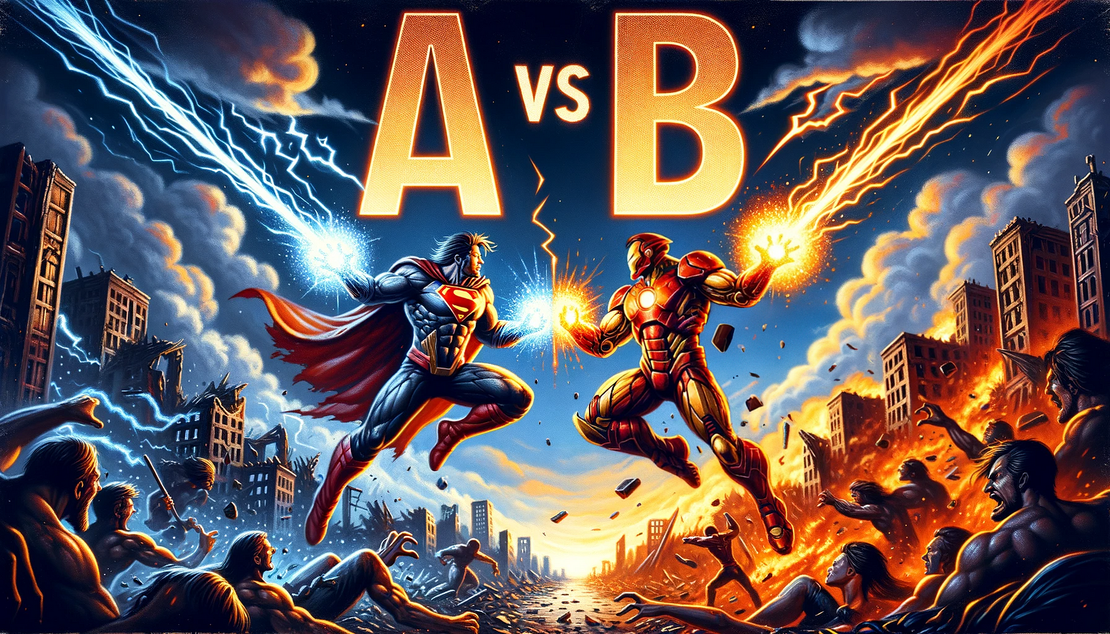
Imagine spending over a decade embedded in a world where every pixel, every sentence, and every call to action can mean the difference between a conversion and a lost opportunity. That’s been my reality for a large part of my career, working at companies that invested hundreds of millions in advertisements, all hinging on the powerful yet delicate process of A/B testing. This journey has provided me with an intuitive feel for what works and what doesn’t, insight that can only be achieved by witnessing the process firsthand.
My experience with Conversion Rate Optimization (CRO) has taught me it’s critical to address some common conversion rate issues before you even begin A/B testing — I’ve detailed some of the more common conversion rate issues in my article: How to Improve Conversion Rates for Your E-Commerce Store: Why Isn’t My Landing Page Converting? By following these initial steps, you’ll be able to harness the power of A/B testing and reap the rewards of your optimization efforts.
What is A/B Testing?
You might have found yourself in a room, sipping on a cup of coffee, while your eyes dart around a screen. You might have asked yourself, “How can I be sure that this button, this sentence, this image is truly what my audience desires?” With A/B testing, the question isn’t “why,” but rather “which one.” Put simply, A/B testing (also referred to as split testing or multivariate testing) is a method of comparing two versions of a web page (or email, marketing copy, etc.) to determine which one performs better. A/B testing can help you optimize your website or app for conversions, such as sign-ups, purchases, downloads, or any other goal you have.
A/B Testing Roadmap (TL;DR)
- Define your goal and hypothesis. What are you trying to achieve with your test and what is your prediction of the outcome?
- Choose your metrics and tools. How will you measure the success of your test and what tools will you use to run and analyze it?
- Select your audience and sample size. Who will participate in your test and how many people do you need to reach a statistically significant result?
- Create and launch your variations. What are the different versions of your web page or app that you want to test and how will you implement them?
- Monitor and analyze your results. How will you track the performance of your variations and compare them to each other and to your baseline?
- Draw conclusions and take action. What did you learn from your test and how will you apply it to improve your website or app?
The world of A/B testing is filled with discovery and continuous improvement. By following these steps, you can be confident in your ability to make informed, data-driven decisions that will ultimately improve your products, benefit your users, and achieve your business goals.
If you’re truly curious to learn more, read on for an in-depth guide to A/B Testing.
Defining Your Goal and Hypothesis
Setting a Clear, Measurable Goal
Before you start A/B testing, it’s vital to have a tangible, quantifiable goal in mind. This goal should be associated with a hypothesis — an educated prediction of the outcomes based on the tweaks you make. Imagine you want to enhance the click-through rate of your email campaign. Your hypothesis could then be that incorporating a personalized subject line will be the catalyst for improvement.
Constructing a Hypothesis
The end goal of creating an A/B test hypothesis is to identify quickly what will help guarantee you the best results. After identifying your business goals, the next step is to generate A/B Testing ideas and hypothesis for why you think they will be better than the current version. You can create a list of all hypotheses that you think you can create, prioritize all variations in terms of the expected impact and how to implement them using various tools.
Choosing Your Primary Metrics
As we venture into the world of A/B testing, one of the fundamental aspects is choosing your primary metrics. It’s a straightforward process — focus on what needs improvement. More often than not, this is something that directly propels revenue. Yet, depending on your product, it could also be a move that boosts engagement or retention, ultimately driving revenue growth.
Aims Behind Picking Metrics
Begin by juxtaposing your marketing campaign outcomes with your quarterly or yearly objectives. Which strategies are falling short? What larger goals is your team struggling with? Let’s say, on analyzing Q2 performance, you find your daily newsletter is scoring an open rate of 15% rather than the intended 30%. This reveals an opportunity for A/B tests to optimize subject lines or email copy — anything that can enhance customer engagement.
Typically, the prime metrics used in A/B testing include conversion rate, click-through rate (CTR), and bounce rate. Conversion rate gauges the occurrence of a desired action, such as an email sign-up or a purchase. CTR measures the clicks received by a digital asset relative to its exposure, while bounce rate reveals the visitors leaving your website without interaction.
Benefits and Goals of A/B Testing
A/B testing not only offers insight into your audience’s behavior but also helps steer your future marketing goals. Here are some objectives marketers typically aim for with A/B testing.
- Increasing Website Traffic: A/B testing can help you pinpoint the highest converting web page titles, drawing your audience’s attention. Experimenting with blog or web page titles can alter the click-rate on these links, consequently driving website traffic. Ever hear the term “clickbait”?
- Boosting Conversion Rates: A/B testing can help increase conversion rates. Tests on varying locations, colors, or texts for your calls-to-action (CTAs) can affect the number of clicks, subsequently increasing the volume of form submissions, contact information, and lead conversions.
- Decreasing Bounce Rate: If visitors are quickly leaving your website, A/B testing helps identify the culprits. Experimenting with blog introductions, fonts, or images can help retain visitors.
- Optimizing Product Images: Ensure you’re presenting your product or service in the most enticing manner by A/B testing your product images. Pick the image that resonates best with your audience based on conversion or click-through rates.
- Reducing Cart Abandonment: With a startling average of 70% of customers abandoning their carts, e-commerce businesses can use A/B testing to improve check-out page designs, product photos, or shipping cost displays to combat this issue.
Identifying the Ideal A/B Testing Solution for You
Selecting an A/B testing software isn’t a one-size-fits-all decision — it depends on your unique needs and constraints. As you prepare to embark on this journey, ask yourself, “What kinds of tests am I planning to run?”. Your answer to this question will act as your compass, guiding your choice of tool.
Considerations for Selecting an A/B Testing Tool
Here’s what you should consider when making your decision:
- Tool Features: Ensure the tool you’re considering aligns with your requirements. Does it possess the specific features needed for your tests?
- Expertise Level: Evaluate the learning curve involved. Is the tool user-friendly or does it require advanced expertise?
- Integration Capabilities: Verify if the tool integrates seamlessly into your existing software ecosystem (e.g. Shopify, WordPress, etc.)
- Pricing and Scalability: Determine if the tool is budget-friendly and whether it can scale with your business growth.
- Reviews and Comparisons: Conduct thorough research by reading reviews and comparing features across multiple tools.
- Trial Period: Take the tool for a spin before fully committing. Most software solutions offer trial periods for this purpose.
- Customer Support and Training: Prioritize tools that provide solid customer support and training options.
If you’re a Blixo customer, reach out to us. We’re here to help you set up A/B testing.
A Few Noteworthy A/B Testing Tools
While I have built in-house tools at previous startups for complete control over the process, I also want to acknowledge some popular external A/B testing and product experimentation tools: Google Optimize, Mutiny, and Optimizely are few worthy mentions that I recommend. Remember, your decision should be based on your specific testing needs and the tool’s ability to fulfill them effectively.
Determining Your Audience and Sample Size for A/B Testing
When conducting A/B testing, you need a significant amount of audience members to ensure that your results are statistically reliable. As a benchmark, I recommend a sample size of at least 1000.
The Essence of A/B Testing
A/B testing or split testing is a straightforward experiment. You divide your audience into two parts and present them with two variations of your marketing content — Version A for one half, Version B for the other. If there is a clear winner, you move forward with the winner and test a new hypothesis.
The Math Behind Sample Sizes
The ideal sample size for your A/B test is not arbitrary. It depends on your expected effect size, significance level, power, and baseline conversion rate. Remember, larger sample sizes usually provide more accurate and reliable results.
However, too small a sample size can skew your results and, consequently, your conclusions. So, you need to ensure that your sample size is adequate to yield accurate results.
To calculate the required number of interactions or visitors for your test, you can use the following formula:
Sample Size = (Z-score^2 * p * (1-p)) / (Minimum Detectable Effect^2)
Here’s what these terms mean:
• Z-score: It represents the number of standard deviations a given proportion is away from the mean. For a 95% confidence level, this would be 1.96.
• p: This represents your expected baseline conversion rate in decimal form (e.g., 3% would be 0.03).
• Minimum Detectable Effect: This is the smallest change in conversion rate you aim to detect, expressed as a decimal (e.g., 20% would be 0.2).
So, let’s calculate:
If we assume a statistical significance of 95%, a baseline conversion rate of 3%, and a minimum detectable effect of 20%, using the formula above, your sample size per group for the A/B test would be approximately 1,068.
Common Pitfalls to Avoid
When selecting your sample size for an A/B test, there are a few mistakes you should avoid:
- Using a Biased Sample: A/B testing relies on random sampling. Bias can easily skew the results.
- Choosing an Insufficient Sample Size: This could lead to unreliable results and may compromise the integrity of your testing process.
- Prematurely Ending the Test: Stopping a test as soon as it achieves the desired confidence level often means your sample size was too small, making the results invalid. Stick to your predetermined sample size to ensure validity.
If you keep these principles in mind, you’ll be well on your way to running a successful A/B test.
Crafting and Launching Your A/B Test Variations
Starting the A/B Testing Process
A/B testing begins with the creation of two variations (Variation A and Variation B) of your website, app, email, or ad content. You distribute these variations equally among your audience and analyze the performance over a significant period.
Establish your control scenario, i.e., the original version of whatever you’re testing. This could be your existing web page or standard landing page design and copy. Then, create your challenger — the modified version that you’ll test against your control. For instance, if you want to determine the effect of adding a testimonial to a landing page, set up your control page without testimonials and include them on your challenger.
However, avoid testing more than one variable at a time, as it can muddy your results. For example, if you concurrently A/B test an email campaign and the landing page it directs to, it becomes challenging to pinpoint which change triggered an increase in conversions.
Strategically Selecting Test Elements
Target elements that likely affect your audience’s engagement with your ads or website. Begin by identifying the elements influencing your sales or lead conversion and prioritize them for testing. You might try different fonts or images for an ad, or contrast two pages to figure out which one keeps visitors engaged longer. Be sure your chosen elements are suitable and modifiable for your testing purposes.
Timing and Scheduling Your Tests
Keeping the rest of your controls consistent, including your testing schedule, is crucial when comparing variables. Make sure to run both variations simultaneously and for the same duration to yield the most accurate results. Choose a time frame that would likely draw similar traffic levels for both parts of your split test.
Remember, different timing variables can influence your campaign results significantly. So, unless you’re explicitly testing optimal timing (such as the best time for sending emails), ensure that variations A and B run simultaneously to avoid ambiguity in your results.
Test One Variable At a Time
Your A/B tests should examine one element at a time to produce reliable results. By doing so, you can determine the specific impact of each variable on consumer behavior. As you progress in optimizing your web pages and emails, you’ll find multiple variables to test. But remember to isolate one independent variable at a time to discern its effectiveness.
Although multivariate testing (testing multiple variables at once) might seem appealing, it requires a massive amount of traffic to be effective, something not every business possesses. If you’re unsure whether to run an A/B test or a multivariate test, stick to the A/B test.
Allowing Time for Meaningful Data
Ensure that your A/B test runs long enough to gather a substantial sample size, leading to statistically significant results. The exact duration varies and is contingent on your company and your website’s traffic levels. The next section will cover how to calculate this.
Gathering Feedback from Users
While quantitative data is valuable, don’t overlook the importance of understanding why consumers behave as they do. Collect qualitative feedback from real users during your A/B test using surveys or polls. These insights can give you a comprehensive understanding of user behavior, complementing the statistical findings of your A/B tests.
Analyzing Your A/B Test Results
Here’s a step-by-step guide to making sense of your A/B test results.
Step 1: Review Test Outcomes
Once you’ve gathered enough information, it’s time to examine it. Your results may depict that either variation A or B has outperformed, or they might be too close to call a winner. Use relevant reports like those available in Google Analytics to compare the results. Depending on your goals, customize these reports to present the information you need.
Step 2: Validate Your Results with an A/B Test Significance Calculator
When analyzing A/B test outcomes, we aim for statistical significance. This means your results aren’t merely random or left to chance. But how do you ensure this? The solution lies in mathematical formulas and tools such as an A/B Test Significance Calculator (most A/B testing solutions have this built in). This formula helps determine if the conversion rate difference between two variations is statistically significant.
Here is a formula for calculating the p-value, which is used to determine the significance level of an A/B test:
p-value = 2 * (1 - norm.cdf(abs(z-score)))
Where:
• norm.cdf is the cumulative distribution function of the standard normal distribution.
• z-score is calculated as (pA - pB) / sqrt((pA * (1 - pA) / nA) + (pB * (1 - pB) / nB))
– pA and pB are the conversion rates of variations A and B, respectively.
– nA and nB are the sample sizes of variations A and B, respectively.
The significance level is calculated as 1 - p-value
. If the significance level is greater than or equal to the desired confidence level (e.g., 95%), then the difference in conversion rates between the two variations is considered statistically significant.
Here are three example scenarios using the formula for calculating the p-value and significance level of an A/B test:
Example 1: Variation A Wins
• Variation A: 1000 visitors, 100 conversions
• Variation B: 1000 visitors, 50 conversions
• Confidence level: 95%
In this scenario, the conversion rates for variations A and B are 10% and 5%, respectively. The z-score is calculated as (0.1 - 0.05) / sqrt((0.1 * (1 - 0.1) / 1000) + (0.05 * (1 - 0.05) / 1000)) = 3.16
. The p-value is calculated as 2 * (1 - norm.cdf(abs(3.16))) = 0.0016
. The significance level is calculated as 1 - 0.0016 = 0.99
(~99%).
Since the significance level is greater than the desired confidence level of 95%, the difference in conversion rates between variations A and B is considered statistically significant. In this case, variation A wins.
Example 2: Variation B Wins
• Variation A: 1000 visitors, 50 conversions
• Variation B: 1000 visitors, 100 conversions
• Confidence level: 95%
In this scenario, the conversion rates for variations A and B are 5% and 10%, respectively. The z-score is calculated as (0.05 - 0.1) / sqrt((0.05 * (1 - 0.05) / 1000) + (0.1 * (1 - 0.1) / 1000)) = -3.16
. The p-value is calculated as 2 * (1 - norm.cdf(abs(-3.16))) = 0.0016
. The significance level is calculated as 1 - 0.0016 = 0.99
(~99%).
Since the significance level is greater than the desired confidence level of 95%, the difference in conversion rates between variations A and B is considered statistically significant. In this case, variation B wins.
Example 3: Not Statistically Significant
• Variation A: 100 visitors, 5 conversions
• Variation B: 100 visitors, 6 conversions
• Confidence level: 95%
In this scenario, the conversion rates for variations A and B are both approximately equal to each other at around ~5%. The z-score is calculated as (0.05 - 0.06) / sqrt((0.05 * (1 - 0.05) / 100) + (0.06 * (1 - 0.06) / 100)) = -0.41
. The p-value is calculated as 2 * (1 - norm.cdf(abs(-0.41))) = 0.68
. The significance level is calculated as 1 - 0.68 = 0.32
(~32%).
Since the significance level is less than the desired confidence level of 95%, the difference in conversion rates between variations A and B is not considered statistically significant.
Step 3: Prioritize Your Goal Metric
Though you’ll measure multiple metrics, your analysis should hinge on your primary goal metric. For instance, if your primary metric is customer purchasing a product, don’t get side-tracked by the click-through rates. Always remember, high click-through rates might not necessarily translate into better conversions.
Step 4: Assess Conversion Rates
An initial glance at the results can reveal which variation performed better. However, you need to determine if the results are statistically significant to claim success. For example, if variation A has a slightly higher conversion rate than B, but the results are not statistically significant and you can’t confidently claim that A will improve your overall conversion rate.
Step 5: Segregate Your Audience
Regardless of your test’s significance, dissecting results based on audience segments is always beneficial. It helps understand how different segments reacted to your variations. Common variables for segmenting audiences include visitor type (new vs. repeat), device type (mobile vs. desktop), and traffic source.
Making Sense of Your A/B Test Results and Next Steps
The end of your A/B testing journey doesn’t just lie in data collection and analysis, but in deriving actionable insights and making those improvements. Here’s how you can turn your data into decisions and what to do next.
Step 1: Analyze the Results
A/B testing enables you to make data-driven decisions, eliminating long arguments about what variation will perform better. Once you’ve thoroughly analyzed the results, identify the superior variation and leverage this data to refine your website or app. If one landing page version outperforms the other, it’s time to update your page with the winning design.
Step 2: Implement Changes
With a clear winner, you can confidently disable the inferior variation in your A/B testing tool and promote the winner. However, if neither variation significantly outperforms the other, it means your test didn’t influence the results, making it inconclusive. In such a scenario, you can either stick with the original variation or rerun the test with new data derived from the failed attempt. Remember, every A/B test, successful or not, provides valuable insights for future efforts. Let’s say your email marketing A/B tests consistently show that putting a person’s first name in the subject line results in better click-through rates. It would be wise to incorporate this tactic in your future email campaigns.
Step 3: Plan the Next Test
Don’t get too comfortable! There’s always room for further optimization. You can even run an A/B test on another aspect of the same web page, app feature, ad, or email you recently tested. If you’ve just tested a landing page headline, consider doing a new test on the body copy, color scheme, or images. Continually be on the lookout for ways to boost conversion rates and leads.
A/B testing is not just about testing and analyzing, but about making informed decisions based on outcomes. It’s a cycle of constant learning and optimizing that guides your business to superior results.